Small is the New Big: The Rise of Small Language Models (SLMs)
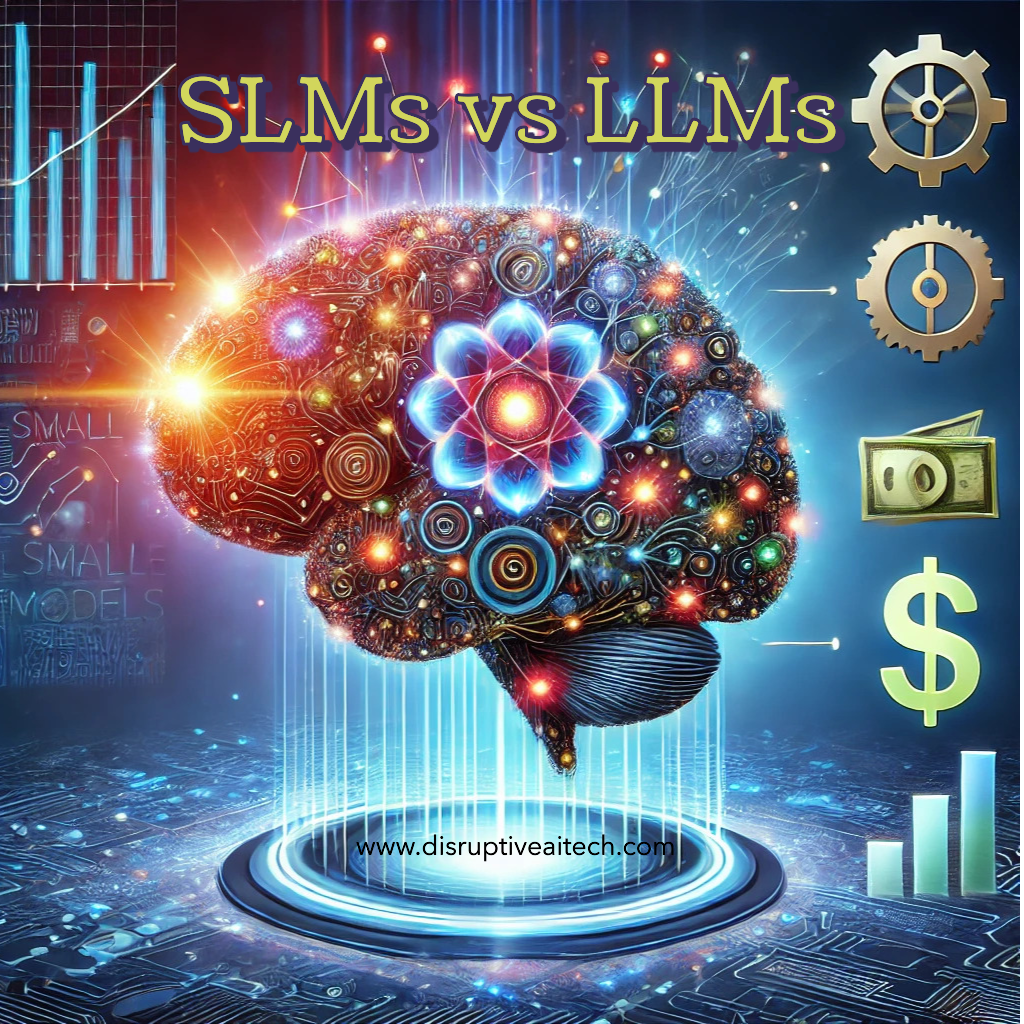
Small language models (SLMs) represent a major change in the field of artificial intelligence since businesses are more and more emphasizing on building small yet effective models. Though giants like GPT-4 have been making news with their remarkable powers, a quiet revolution is under way in the field of what we refer to as Small Language Models (SLMs). These little powerhouses show that, in artificial intelligence, larger isn’t always better. Let’s delve into the realm of SLMs and investigate why they are the buzz of the tech scene.
Designed to be highly performing at a fraction of their larger equivalents, these models—OpenAI’s GPT-4o small and Mistral’s NeMo 12B—are The most recent advancements in SLMs, their affordability, and their future promise are investigated in this blog.
What are Small Language Models?
As their name would imply, little language models are scaled-down variants of the big language models we know. Still keeping a high degree of performance for particular jobs, they are meant to be more efficient, faster, and more accessible. Among the notable ones are:
- GPT-4 Mini: OpenAI recently launched GPT-4o mini, a streamlined version of its flagship model, GPT-4o. This model is priced at 15 cents per million input tokens and 60 cents per million output tokens, making it over 60% cheaper than the previous GPT-3.5 Turbo model. Despite its smaller size, GPT-4o mini boasts impressive capabilities, scoring 82% on the MMLU benchmark, which surpasses competitors like Google’s Gemini Flash and Anthropic’s Claude Haiku.
- Mistral AI’s Models: In collaboration with NVIDIA, Mistral AI introduced the NeMo 12B, a small language model with 12 billion parameters. This model is designed for various applications, including chatbots and coding tasks, and excels in reasoning and world knowledge. NeMo also features:
- 128K token context window, allowing for extensive input handling
- State-of-the-art performance in multi-turn conversations and mathematical reasoning
- Open-source availability under the Apache 2.0 license, promoting widespread adoption and customization
3. Other Notable SLMs:
Additional models like Hugging Face’s SmolLM and Mistral’s specialized models (Mathstral for STEM reasoning) are also gaining traction. SmolLM comes in various sizes (135M to 1.7B parameters) and has shown strong performance in common sense reasoning tasks. These innovations highlight a growing trend toward developing smaller, specialized models that cater to specific needs.
Why are Companies Developing SLMs?
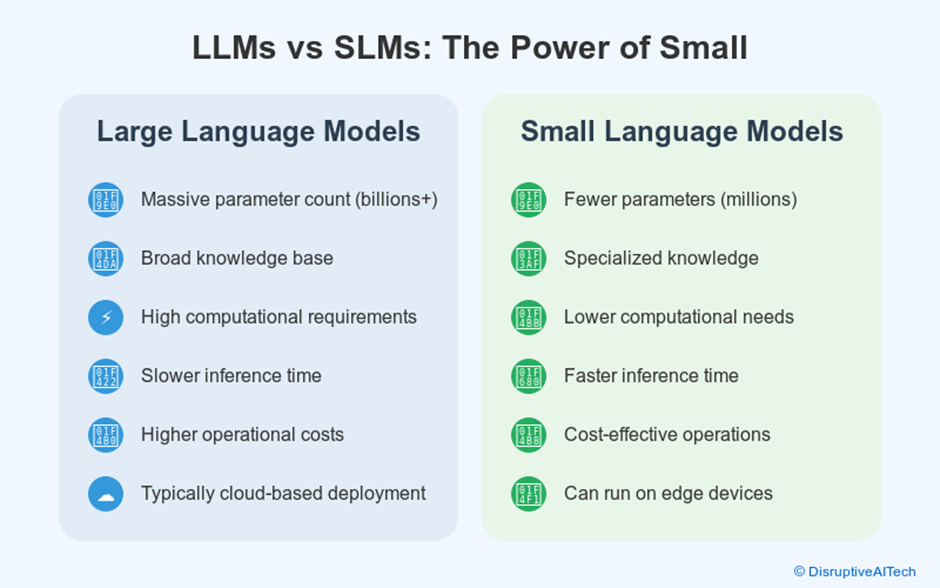
Cost Efficiency
The development of SLMs was driven mostly by their cost-effectiveness. Businesses can include strong AI capabilities without paying outrageous fees since operational expenses are far lower than those of larger models. GPT-4o Mini’s pricing approach, for example, lets developers and businesses both utilize it widely, therefore democratizing access to artificial intelligence technologies.
Accessibility
SLMs are meant to be more easily available to a larger population. Companies let developers—from startups to big businesses—use artificial intelligence without significant infrastructure investments by lowering costs and streamlining integration via APIs. From customer service to education, this accessibility encourages invention in many different fields.
Performance Without Compromise
SLMs, for all their diminutive scale, never compromise performance. Their better architectures and training approaches help them to often outperform more general models in certain tasks. For coding accuracy and logic, for instance, both GPT-4o small and Mistral NeMo have shown better performance than their more ambitious successors.
Small Language Models: The Future
As they develop, SLMs seem to have bright future. Many patterns point to the direction this technology is going:
Increased Specialization: As companies recognize the value of tailored solutions, we can expect more specialized SLMs that excel in niche applications—such as STEM education or multilingual support—further enhancing their utility in specific industries.
Wider Adoption: With ongoing advancements in training techniques and hardware capabilities, SLMs are likely to see broader adoption across various sectors. Their affordability will make them attractive options for businesses looking to implement AI solutions without significant upfront investments.
Integration with Emerging Technologies: Future iterations may integrate seamlessly with other emerging technologies like augmented reality (AR) and virtual reality (VR), expanding their application scope beyond traditional text-based tasks.
Open-source Movement: The trend towards open-source models will likely continue, fostering community-driven innovation and allowing organizations to customize AI solutions according to their specific needs.
The rise of Small Language Models represents a significant shift in the AI landscape. By proving that efficient, specialized models can often outperform their larger counterparts in specific tasks, SLMs are challenging our assumptions about what’s possible in AI. As companies continue to innovate in this space, we can look forward to a future where AI is not just more powerful, but also more accessible, efficient, and tailored to our needs.
The old adage “good things come in small packages” has never been more true in the world of AI. As we move forward, it’s clear that in the realm of language models, small is indeed the new big.
References:
1. https://www.learngrowthrive.net/p/mistral-nemo-12b-gpt4o-mini-llama-3
4.https://www.linkedin.com/news/story/openai-launches-gpt-4o-mini-6829946/